Battery Material Characterisation and Digital Twins for Cell to Pack Performance in Agile Manufacturing Pilot Lines and Automotive Field
Website: https://www.digicell-project.eu/
Abstract:
Reliability and innovations in current and upcoming battery technology as one core element of Europe’s green industrial transition are highly dependent on the understanding and systematic classification of the complex processes in advanced functional materials structured at the multiscale level.
DigiCell provides a digitally integrated framework that improves reliability and quality in the manufacturing processes of high-performance Lithium-ion batteries (LIB) and beyond Lithium battery technologies through unified and adaptive models capturing the structure-property relationships in these complex energy materials. It is based on a toolset of innovative and state-of-the-art characterisation methods for multiscale materials, interoperable tests, and analytical models supported by and linked through machine learning. With this, the production costs, materials waste, and the CO2 footprint in production lines will be reduced, while in parallel the battery electrochemical performance at the single cell level will be increased. The new measurement tools and multi-scale modelling algorithms lead to a higher characterisation speed (factor of 5) and an improved accuracy in cell tests by an order of magnitude, as will be demonstrated on the lab bench and in pilot lines. DigiCell develops a new holistic approach for open-source algorithms and data standardization strategies; new quality assessments for a healthy, safe, and circular economy. The project readily interfaces and interacts tightly with EMMC.
Leitat’s Role in the Project:
Leitat’s primary role and contribution to the project focuses on Combined AI forecasting with ma-DMP including inline sensors and electric characterisation for agile cell manufacturing, Nanoscale electrochemical imaging for the characterisation of electrode materials and interface layers, Evaluation of the effect of electrolyte aging and electrode consumption on the battery performance, AI/ML and FEM forecast modelling of electrochemical materials, linking multiple physics and scales, Manual and automated data acquisition methods by developing a prediction model based on aging test data.
Project Budget: 5,405,098.00€
Leitat Budget: 425,442.50€
Financial Framework: HORIZON EUROPE
Contract number: 101135486
Start Date: 01/01/2024
End Date: 31/12/2026
Partners:
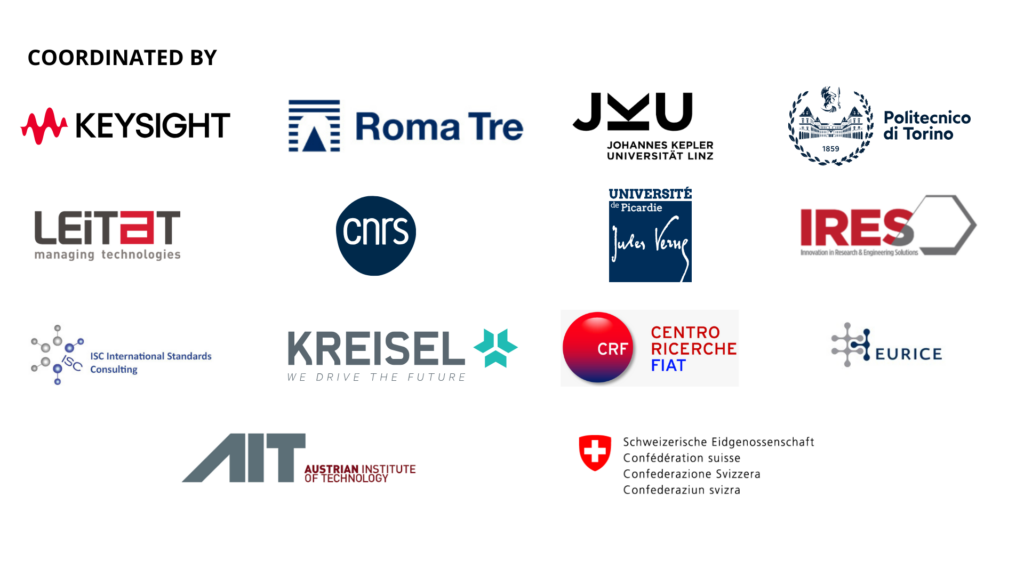
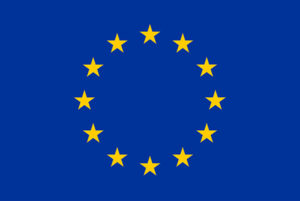
This project has received funding from the European Union’s Horizon Europe research and innovation programme under grant agreement No 101135486. Funded by the European Union.